Emerging Trends in Artificial Intelligence for Multisystem Diagnosis
- Journal of Empirical Reviews in Multisystem Syndromes. JERMS
- Nov 6, 2024
- 5 min read
Journal of Empirical Reviews in Multisystem Syndromes (JERMS)
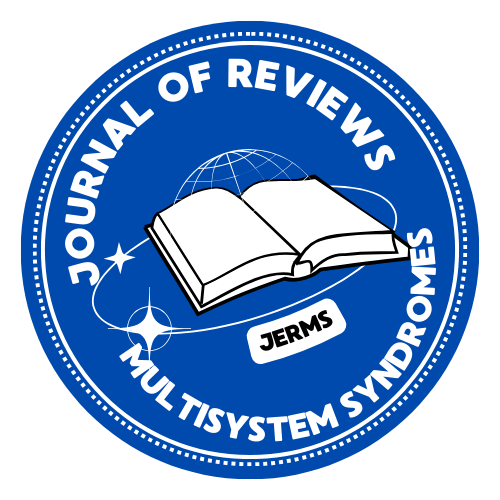
Galy Masm: Editorial TeamMember
Published: Nov/2024
Vol. 1, Issue 1
The rapidity of developments within the past decade in artificial intelligence and machine learning is an influence that extends from finance to transportation. In health care, these technologies hold transformative potential, particularly in the study and management of multisystem syndromes. The difficulty in treating multisystem diseases often lies in the fact that symptoms can manifest in a variety of forms across numerous organ systems. This may result in fragmented or delayed care, as the traditional diagnostic methods cannot connect symptoms that seemingly appear disparate. However, AI and ML are emerging tools that hold great promise for addressing these challenges by analyzing large datasets, picking out patterns that elude conventional analysis, and generating insights to inform more precise, timely, personalized approaches to managing complex diseases.
The Journal of Empirical Reviews in Multisystem Syndromes thus finds the increasing and appropriate participation of AI in health, especially regarding diagnosis that involves multisystems. We aim to further enable knowledge related to the use of AI in the management and early identification of various disorders affecting different organ systems by investigating the role of technology at the very core of medicine. This editorial will outline current and potential applications of AI in multisystem diagnosis, remaining challenges and limitations, and implications for the future of multisystem syndrome research.
The Promise of AI in Multisystem Diagnosis
Multisystem syndromes-such as systemic lupus erythematosus, chronic fatigue syndrome, and post-acute sequelae of SARS-CoV-2 infection, better known as "long COVID"-are notoriously difficult to diagnose. Many of them are systemic disorders that involve various systems like the neurological, cardiovascular, and musculoskeletal, thus creating a real diagnostic challenge, which may sometimes be unreachable by traditional means. Diagnosis is also often delayed or completely missed because these syndromes may present in similar ways as many other conditions that are more common. Solutions to these challenges provided by AI and ML will be through the processing and analysis of extensive data on symptoms, lab results, imaging, and patient histories to enable the detection of subtle patterns indicating multisystem involvement.
Pattern Recognition: One of the strong points in the use of AI in medicine is its capacity for pattern recognition. Because of this, AI algorithms can analyze data on a scale and speed unmatched by humans, finding patterns and correlations that otherwise might never be seen. For instance, AI systems can analyze thousands of patient records in their search for commonalities among people having similar symptoms, even though the symptoms are spread out over different systems. This ability can also help in identifying "hidden" connections and thus lead to early detection of multisystem syndromes, perhaps even before symptoms have developed to severe or disabling levels. Early identification not only improves the accuracy of diagnosis but also opens opportunities for early intervention that may be critical in conditions with progressive symptoms or irreversible damage.
Predictive Analytics: AI has enormous potential to predict the course of a disease in multisystem syndromes. Applying machine learning models against longitudinal data derived from similar syndrome patients, healthcare professionals are now in a position to make intelligent predictions about the likely course of disease a patient will follow. For example, in systemic autoimmune diseases like lupus, AI may use historical data to predict complications that may arise in different systems, such as cardiovascular or renal involvement. Predictive models have a great value in multisystem conditions, enabling proactive monitoring and management of the patients, hence reducing the risks of severe complications.
Data Integration: Probably the toughest part of multisystem diagnosis is integrating data coming from multiple sources, including clinical notes, lab results, imagery studies, and even wearable health monitors. AI will contribute to making this easier by pulling in data from a variety of sources and building one unified comprehensive view of a patient's health. With recent enhancements in NLP, the algorithms can now even analyze unstructured data-such as physician notes-to find symptoms and insights that may not be immediately apparent from the structured data alone. This integration is invaluable for multisystem syndromes, where nonspecific symptoms can be significant indicators when combined with data from other systems.
Examples of AI Applications in Multisystem Syndrome Research
Applications of AI in multisystem diagnosis are highly promising and already find realization in several research and clinical applications:
Autoimmune Diseases: AI algorithms have been used to analyze biomarkers of the immune system that are related to lupus, rheumatoid arthritis, and other autoimmune diseases, enabling the recognition of patterns leading up to disease flares. Machine learning models may be trained on imaging and lab data in order to differentiate subtypes of the disease, therefore allowing for precision diagnosis and individualized treatment.
Cardiovascular-Neurological Interactions: Recent studies are employing AI to explore connectivity between cardiovascular and neurological manifestations of long COVID. For example, some use ML to investigate ECG data in parallel with brain imaging data, seeking patterns that correspond to cardiac symptoms and overall cognitive symptoms. This kind of approach will thus give a more holistic insight into the syndrome itself and help targeted interventions.
Multisystem rare diseases represent an area where AI is quite useful, since diagnostic criteria are not often established, and clinical databases might be underpopulated. AI, in this respect, stands a better chance of analyzing the genetic, clinical, and imaging data for possible markers of rare multisystem diseases and helping their diagnosis at an early stage when symptoms can seem nonspecific.
Challenges and Limitations of AI in Multisystem Diagnosis
While applications of AI to multisystem diagnosis are promising, there are still some challenges that remain, such as data quality and availability: for any effective AI, high-quality data is required, emanating from large and diverse patient populations. Regrettably, the data concerning multisystem syndromes is slender, with many conditions being mis- or underdiagnosed. Besides, there is considerable inconsistency in healthcare data between systems, further complicating the creation of reliable AI models.
Interpretability: Amongst the major criticisms of AI, especially in medical health, it can be said that a lot of algorithms are "black box" in nature and impede detailed understanding of its decision-making processes. In multi-system syndromes where symptoms and outcomes are so highly individualized, interpretability of AI outputs by clinicians for allowing informed decisions could well be explained to patients.
Ethical Issues: AI also raises a number of ethical issues within the field of medicine-specifically, data privacy, which is highly sensitive with regard to health. Since most AI models are built upon historical data, there is a real possibility of propagating the already existing biases of health care. These concerns need to be addressed if one wants trustworthy and fair AI systems.
Positioning JERMS at the Forefront of AI-Driven Multisystem Research
The Journal of Empirical Reviews in Multisystem Syndromes is committed to such research efforts involving AI and machine learning for further understanding and management of the diseases. Therefore, JERMS would like to contribute to the evolving landscape of multisystem disease management by providing an avenue for interdisciplinary and technologically forward-looking research.
We invite submissions, especially on AI-driven methods of data integration, predictive modeling, and pattern recognition in the scenario of multisystem conditions. Publishing research on the applications of AI in multisystem syndromes, JERMS aims at moving the field forward to promote a new frontier of diagnostic and therapeutic innovation. We are committed to facilitating discussions between healthcare providers, data scientists, and patients by inviting research that is scientifically rigorous and ethically sound.
Conclusion: AI integrated into multisystem syndromes research epitomizes a very promising frontier in healthcare. As the challenges of multisystem conditions continue to test traditional approaches, involvement in diagnosis and management by AI will most likely increase, offering new ways of understanding, prediction, and treatment of these conditions.
Comments